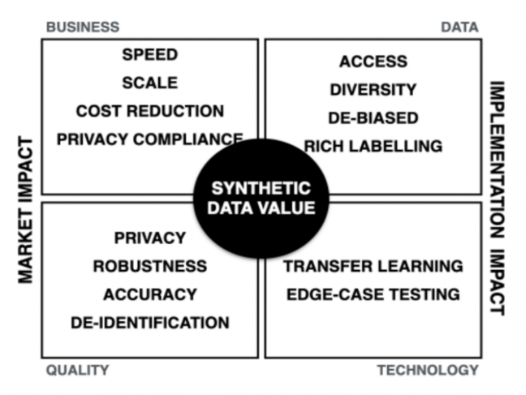
Interest in synthetic data has increased in the last few years. In a recent report Gartner predicts that by 2024, 60% of data used for the development of AI solutions will be synthetically generated. But can synthetic data successfully address the data challenge that is holding back the widespread adoption of AI?
The appeal of synthetic data is widespread – in terms of utility and privacy, it can generate a more diverse dataset, cover edge conditions that are difficult to collect, and meet privacy expectations.
In this white paper, we look at synthetic data in-depth and explore questions on its need, application, challenges and potential. We share our early experience and learnings through a case study that describes the synthetic data generation method that we used to generate data and the process applied to train a computer vision model that detects traffic signage for safety compliance for utilities.
Our initial work has set the foundation to further build the synthetic data pipeline. It has also highlighted existing gaps and areas of further study. We have seen that synthetic data is an effective method to supplement small data sets, and it provides an option to demonstrate day-one AI value for niche vertical enterprise applications.
The role of synthetic data will continue to evolve and has the potential to cut down the volume of real-world data required – a victory in itself. Beyond that, any gain that we can deliver is an added bonus. Trust in the generated data is still developing and will remain a critical factor for the adoption of data synthesis. It will be interesting to see how this evolution unfolds and the time it takes to make a noticeable impact.
Explore synthetic data, its applications, challenges and more in this white paper.