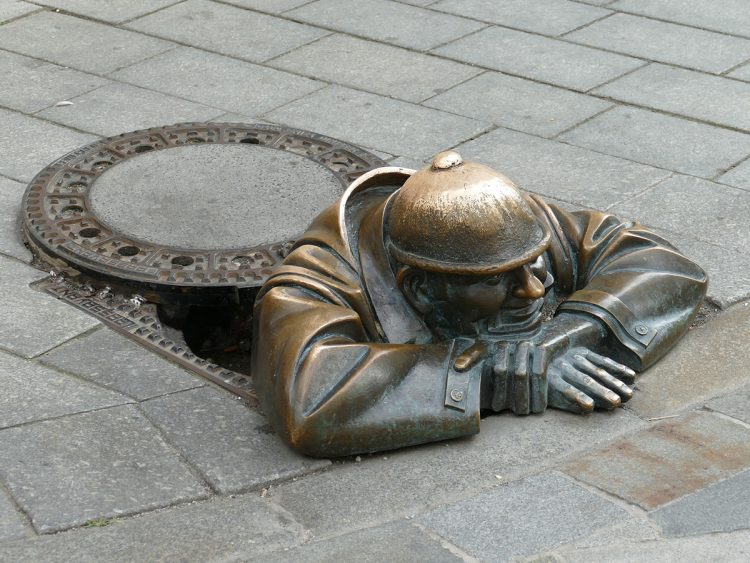
The wrong implementation of data science can have negative consequences for a company. Our advisor Mayank Sharma is an expert in the field, and he shares several best practice tips for implementing a successful data science initiative.
When data science goes wrong – how to reduce risk
Unfortunately, businesses that are under competitive stress often implement a data science agenda prematurely. They quickly see a negative impact on customer satisfaction, quality or revenue. On the other hand, some companies adopt a more thoughtful and measured data science strategy and are thus able to benefit from the power of technology. At the end of the day, business leaders need to make a judgment call on how accurate they wish their algorithms to be for the benefits to outweigh the risks. Here are some ways to reduce that risk:
1. Be skeptical, be realistic
If you have attended numerous technical sales presentations and they sound all too similar, it’s ok. It’s not you, it’s them. Data Science is a hot area and has spawned many pretenders that purport to do the same things with the same technologies and the same ideas. If you’re promised that all your data will be magically ingested, digested and turned into gold – you should put on your doubter’s hat. Ask probing questions, try to ascertain if the technology provider has domain expertise in your industry and is honest about the potential difficulties inherent in solving your business problems. Retail is not Healthcare is not Finance. Data Science is not magic.
On the other hand, if as a business executive you have begun to feel confident that you understand what data science is about simply because you can recite the story at the input-output level without knowing much about the tricky bits in the middle, then stop. Chances are you don’t understand the crucial elements of a successful data science strategy; and may consequently not be able to tell the difference between a serious technology provider and one that has simply mastered the script. Nor will you be able to assess the true heft, cost and time required for your organizational transformation.
2. Get your ducks in a row
If you have antiquated processes and needlessly complex workflows, fix them first. Data Science can address only so much inefficiency. If your problem is digitalization, don’t rush to AI expecting some panacea. Learn to walk before attempting to run. It is worrisome how many organizations claim that they are using AI but who are actually simply getting around to digitizing their business artifacts.
Just as a person needs context to make a good decision, so do algorithms. You should have a good view across the organisation before building a data science strategy. Too often, data science efforts start with a narrow scope, but if your problem spans your organisation and has external factors influencing it, then knowing just about your local environment is not enough to solve the problem. There are unintended consequences of creating models based on incorrect or incomplete information.
But if you start with a problem that is restricted to one part of an organisation, and it’s localised, that’s fine, because that’s all the context you need to analyse the problem. This is where I recommend you start.
3. To get buy-in from key stakeholders, start small
Management tends to have a reasonable resistance to new ideas – leaders everywhere are averse to losing revenue or performance based on an idea that they’re not totally comfortable with. We are in that transitional phase where people still need to be convinced of the impact that data science can have.
If data scientists come in with the humility to say, “We understand there is complexity, and the prototype that we’ll use to build a pilot is not the same as the finished product. It will take time to get to the end and we will need to work closely with the subject matter experts”, then progress can be made.
You need to prioritise, from amongst a thousand things that you could do, the ones that hit the sweet spot in terms of the amount of data available, the maturity of the field (Are there algorithms available to solve the problem?), and the potential impact that it might have for your organisation.
If you can prove that this one small thing works, and key stakeholders see the value that it brings, then you can grow that program to encompass more and more problems across the organisation.
This is easier said than done, because being thoughtful is not easy. It’s not “cost-effective” in the short term – it takes time.
4. Incentivise long-term planning
Many personal incentives are aligned, unfortunately, to monthly or quarterly performance. Too many people would rather get short-term suboptimal gains because long-term business benefits are not necessarily in their best interest.
Management should keep in place a structure of incentives for long-term planning. This doesn’t have to be twenty years, it could be five years, but it should be more than a month or a quarter.
In the end, data science is a thoughtful and exploratory discipline, and the long-term benefits can be significant compared to the investments you put in today.
This is part 3 of 3 in a blog series called “Demystifying Data Science”. Follow vyn on LinkedIn or Twitter to get more insights like this, or sign up to our newsletter.
Leave a reply